Big Data’s Big Muscle
Finance & Development, September 2016, Vol. 53, No. 3
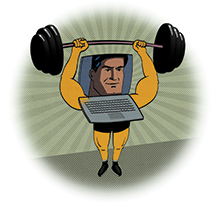
Computing power is driving machine learning and transforming business and finance
The world has access to more data now than was conceivable even a decade ago. Businesses are accumulating new data faster than they can organize and make sense of it. They now have to figure out how to use this massive amount of data to make better decisions and sharpen their performance.
The new field of data science seeks to extract actionable knowledge from data, especially big data—extremely large data sets that can be analyzed to reveal patterns, trends, and associations. Data science extends from data collection and organization to analysis and insight, and ultimately to the practical implementation of what was learned. This field intersects with all human activity—and economics, finance, and business are no exception.
Data science brings the tools of machine learning—a type of artificial intelligence that gives computers the ability to learn without explicit programming (Samuel, 1959). These tools, coupled with vast quantities of data, have the potential to change the entire landscape of business management and economic policy analysis.
Some of the changes offer much promise.
Consumer profiling
The rapid growth in the adoption of data science in business is no surprise given the compelling economics of data science.
In a competitive market, all buyers pay the same price, and the seller’s revenue is equal to the price times the quantity sold. However, there are many buyers who are willing to pay more than the equilibrium price, and these buyers retain consumer surplus that can be extracted using big data for consumer profiling.
Charging consumers different prices based on their analyzed profiles enables companies to get the highest price the consumer is willing and able to pay for a specific product. Optimizing price discrimination or market segmentation using big data is extremely profitable. This practice was the norm in some industries—for example, the airline industry—but is now being extended across the product spectrum.
Moreover, the gains from price targeting also enable firms to offer discounts to consumers who would not otherwise be able to afford the equilibrium price, thereby increasing revenue and expanding the customer base, and possibly social welfare. Consumer profiling using big data is an important reason for the high valuations of firms such as Facebook, Google, and Acxiom, which offer products and services based on their customers’ data.
While big data may be used to exploit consumers, it is also changing business practices in a way that helps those same consumers. Firms are using the data generated from people’s social media interactions to better understand their credit behavior. Relating people’s past credit history to their social media presence leads to improved credit-scoring systems. It also allows lenders to extend credit to people who might otherwise be turned down.
In particular, big data eliminates the biases that arise when people make decisions based on limited information. This absence of fine-grained individual data led to redlining in loan applications, a practice dating to the 1930s. Mortgage lenders would draw red lines around areas on a map to indicate that they would not make loans there because of the racial or ethnic composition. This stereotyping practice denied credit to entire segments of society.
Big data, however, does away with stereotyping. Coarse subjective data can now be replaced by finer, more individualized data. Credit-scoring firms can exploit the heterogeneity detectable from people’s social media interactions, texting streams, microblogs, credit card patterns, and profiling data—in addition to such typical demographic data as income, age, and location (Wei and others, 2014). The use of finer-grained data facilitates better classification of individuals by credit quality.
Forecasting and risk analysis
Economic forecasting has changed dramatically with data science methods. In traditional forecasting, key statistics about the economy—such as the quarterly GDP report—are available only with considerable delay. Data science can get around these delays by relying on information that is reported more frequently—such as unemployment figures, industrial orders, or even news sentiment—to predict those less frequently reported variables.
The collection of approaches engaged in this activity is known as “nowcasting”—also termed the prediction of the present—but is better understood as real-time forecasting (see “The Queen of Numbers,” in the March 2014 F&D).
Data science is also making inroads when it comes to analyzing systemic financial risk. The world is more interconnected than ever, and measuring these ties promises new insight for economic decision making.
Looking at systemic risk through the lens of networks is a powerful approach. Data scientists now use copious data to construct pictures of interactions among banks, insurance companies, brokers, and more. It is obviously useful to know which banks are more connected than others. So is information about which banks have the most influence, computed using a method based on eigenvalues. Once these networks are constructed, data scientists can measure the degree of risk in a financial system, as well as the contribution of individual financial institutions to overall risk, offering regulators a new way of analyzing—and ultimately managing—systemic risk. See Espinosa-Vega and Solé (2010); IMF (2010); Burdick and others (2011); and Das (2016).
These approaches borrow extensively from the mathematics of social networks developed in sociology, and they are implemented on very large networks using advanced computer science models, culminating in a fruitful marriage of several academic disciplines.
More than words
Text analytics is a fast-growing area of data science and is an interesting complement to quantitative data in the area of finance and economics (see “Two Faces of Change,” in this issue of F&D). Commercial applications based on text mining abound: firms like iSentium extract long- and short-horizon sentiment from social media using Twitter; StockTwits provides sentiment indicators through a mobile-enabled Web application.
It is now possible to rank a firm by quarterly earnings outcomes in its 10-K, an annual report on a company’s financial performance filed with the U.S. Securities and Exchange Commission (SEC). A tally of risk-related words in quarterly reports offers an accurate ranking system for forecasting earnings. Firms whose quarterly reports are harder to read tend to have worse earnings—most likely because they attempt to report bad news using obfuscating language (see Loughran and McDonald, 2014). Using an age-old metric for readability, the Gunning Fog Index, it is easy to score financial reports on this attribute, and regulators such as the Consumer Financial Protection Bureau are looking into establishing readability standards.
Studies have even found that the mere length of the quarterly report is sufficient to detect bad news (longer reports presage earnings declines), again because obfuscation is correlated with verbiage; as an ultimate extension, the file size alone of companies’ filings uploaded to the SEC’s website signaled quarterly earnings performance. Much more is expected to emerge from this rapidly evolving area of work.
A new field known as “news analytics” mines the news for data. Services provided by companies such as RavenPack are growing. These services range from sentiment scoring and predictive analytics for trading to macroeconomic forecasting. RavenPack mines vast quantities of unstructured data from news and social media and converts it into granular data and indicators to support financial firms in asset management, market making, risk management, and compliance.
Within this category, news flow analysis is especially interesting. Hedge funds mine thousands of news feeds a day to extract the top five or ten topics and then track the evolution of the proportion of topics from day to day to detect tradable shifts in market conditions. A similar analysis would be useful to policymakers and regulators, such as central bankers. For example, it might be time to revisit interest rate policy when the proportion of particular topics discussed in the news (such as inflation, exchange rates, or growth) changes abruptly.
Topic analysis begins with construction of a giant table of word frequencies, known as the “term-document matrix,” that catalogs thousands of news articles. Terms (words) are the rows of the table, and each news article is a column. This huge matrix can uncover topics through mathematical analysis of the correlation between words and between documents. Clusters of words are indexed and topics detected through the use of machine learning such as latent semantic indexing and latent Dirichlet allocation (LDA). LDA analysis produces a set of topics and lists of words that appear within these topics.
These modeling approaches are too technical to be discussed here, but they are really just statistical techniques that uncover the principal word groupings in a collection of documents (for example, in the news stream). These language clues are likely to be widely used by economic policymakers and in political decision making—for example, in redefining the message in a political campaign.
Artificial intelligence and the future
Computers are more powerful than ever, and their ability to process vast amounts of data has stimulated the field of artificial intelligence. A new class of algorithms known as “deep-learning nets”—inspired by biological neural networks—has proved immensely powerful in mimicking how the brain works, offering many successful instances of artificial intelligence.
Deep learning is a statistical methodology that uses artificial neural networks to map a large number of input variables to output variables—that is, to identify patterns. Information is dissected through a silicon-and-software-based network of neurons. Data are used to strengthen the connections between these neurons, much as humans learn from experience over time. The reasons for the stunning success of deep learning are twofold: the availability of huge amounts of data for machines to learn from and the exponential growth in computing power, driven by the development of special-purpose computer chips for deep-learning applications.
Deep learning powers much of the modern technology the world is beginning to take for granted, such as machine translation, self-driving cars, and image recognition and labeling. This class of technology is likely to change economics and policy very soon. Credit rating agencies are already using it to generate reports without human intervention. Large deep-learning neural networks may soon provide forecasts and identify relationships between economic variables better than standard statistical methods.
It is hard to predict which domains in the dismal science will see the biggest growth in the use of machine learning, but this new age has definitely arrived. As noted science fiction writer William Gibson put it, “The future is already here; it’s just not very evenly distributed.” ■
References
Billio, Monica, Mila Getmansky, Andrew W. Lo, and Loriana Pelizzon, 2012, “Econometric Measures of Connectedness and Systemic Risk in the Finance and Insurance Sectors,” Journal of Financial Economics, Vol. 104, No. 3, pp. 535–59.
Burdick, Douglas, Mauricio A. Hernandez, Howard Ho, Georgia Koutrika, Rajasekar Krishnamurthy, Lucian Popa, Ioana Stanoi, Shivakumar Vaithyanathan, and Sanjiv Das, 2011, “Extracting, Linking and Integrating Data from Public Sources: A Financial Case Study,” IEEE Data Engineering Bulletin, Vol. 34, No. 3, pp. 60–7.
Das, Sanjiv, 2016, “Matrix Metrics: Network-Based Systemic Risk Scoring,” Journal of Alternative Investments, Vol. 18, No. 4, pp. 33–51.
Espinosa-Vega, Marco A., and Juan Solé, 2010, “Cross-Border Financial Surveillance: A Network Perspective,” IMF Working Paper 10/105 (Washington: International Monetary Fund).
International Monetary Fund (IMF), 2010, “Systemic Risk and the Redesign of Financial Regulation,” Global Financial Stability Report, Chapter 2 (Washington, April).
Lin, Mingfen, Nagpurnanand Prabhala, and Siva Viswanathan, 2013, “Judging Borrowers by the Company They Keep: Friendship Networks and Information Asymmetry in Online Peer-to-Peer Lending,” Management Science, Vol. 59, No. 1, pp. 17–35.
Loughran, Tim, and Bill McDonald, 2014, “Measuring Readability in Financial Disclosures,” Journal of Finance, Vol. 69, No. 4, pp. 1643–71.
Samuel, A.L., 1959, “Some Studies in Machine Learning Using the Game of Checkers,” IBM Journal of Research and Development, Vol. 3, No. 3, pp. 210–29.
Wei, Yanhao, Pinar Yildirim, Christophe Van den Bulte, and Chrysanthos Dellarocas, 2015, “Credit Scoring with Social Data,” Marketing Science, Vol. 352, pp. 234–58.
Opinions expressed in articles and other materials are those of the authors; they do not necessarily reflect IMF policy.